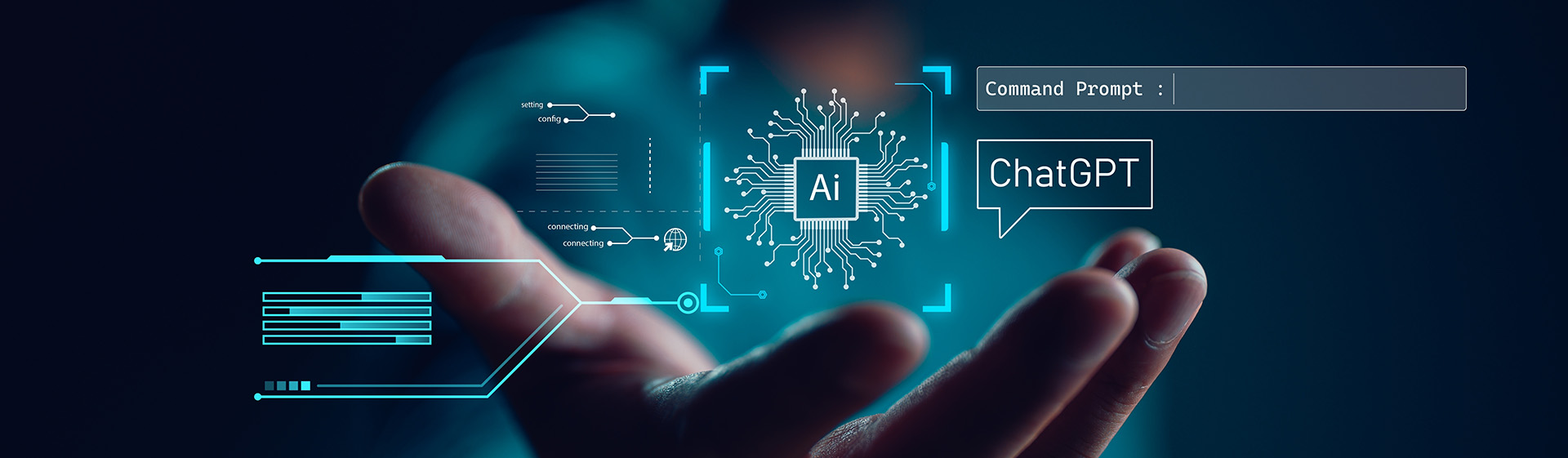
AUTHOR Rob Fell
I bought a new washing machine recently.
From the maker of some of the best consumer electronics that South Korea has to offer, this marvel of modern technology includes an “AI Wash” mode that senses weight load and balance, the amount of washing required, and optimises detergent usage and cycle timing. This is all achieved not via the old generation combined hardware & firmware electronics methods, but with a machine learning AI algorithm that adapts to clothes type, habits, and preferences. As an interesting bonus (the true value of which I’ve yet to truly determine) it is robustly connected to our home Wi-Fi network via a SmartThings app on my ‘phone, so I can even control the cycle and check on the status of the family’s socks from anywhere in the world. Nice.
One of the most powerful commercially available AI engines is provided by Tesla for their FSD autonomous driving application in their range of world beating EVs. This real-time adaptive software is remarkable in its abilities and is currently a long way ahead of its closest rivals in the automotive world.
So, AI is here to stay. But what exactly is it? It seems that there are many emulators but relatively few genuine AI applications.
Amongst his other groundbreaking work, the great Alan Turing created the Turing Test: a method of inquiry in artificial intelligence (AI) for determining whether or not a computer is capable of thinking or reacting as a human being. Turing also provided the following broad definition “AI is the science and engineering of making intelligent machines, especially intelligent computer programs.” But some 50 years have passed since then, and I think that there is a lot more to the story now.
In a vastly simplistic definition, AI applications use an algorithm and dataset to provide the user with a human-like response, define a set of actions or provide a form of data analysis. So far, so good. However, all AI is not created equal, nor will it evolve to achieve equity – and currently there is no one application to unify the outputs of the hundreds of AI models now running (Skynet, anyone?).
It’s true for us to say that “AI machine learning” systems are becoming a part of everyday life, are here to stay, and will continually evolve as their capabilities and knowledge databases grow. That evolution is happening now at an unprecedented rate.
But is it all good? Science fiction certainly teaches us that we should be more than a little wary and a growing number of technical specialists in the real world are increasingly concerned.
The AI label – true AI or limited intelligence?
The two dominant forms of artificial intelligence are termed Narrow AI and Broad AI. Narrow AI is restricted to machine-learning applications and as such it is limited in its reach and capability, so my washing machine is unlikely to take over the world anytime soon. There are things it can do however in terms of revenue generation by reporting back usage and performance data to its maker via the interconnectivity app.
But generative AI is still some way from being sufficiently developed for businesses to rely on its output. The baseline data that is utilised and the way that the AI algorithm applies that data is subject to bias and offset either by design or potentially by a regulatory body. The EU has already adopted the AI Act 2023, and the US isn’t far behind in terms of establishing its own rules on oversight.
There was a publicised case recently involving a lawyer in the US who used ChatGPT to research case precedents for an upcoming hearing. This saved hours of poring over case history books and provided an impressive list of precedents in minutes that could be argued by the lawyer in court. Fantastic, except the case precedents were all fabricated by the generative AI engine because it couldn’t find any real ones.
Red faces all round in the defendant’s camp, then. However, this anecdote highlights the dangers in relying on fixed database generative AI applications for any serious research purpose, and the potential for ‘word salad’ document drafts remain a significant risk.
Applications – everywhere
There has been an explosion in AI applications covering a seemingly inexhaustible list of subjects. AI will write code, Excel functions, modify photographs and generate graphics, generate deep-fake videos, build e-commerce websites, even stock-market trading is covered (with a success rate that easily beats the seasoned floor traders). It’s easy to anticipate how the careful use of an AI application can boost productivity and that translates directly to any company’s bottom line.
Regulators – mount up
Okay, so not exactly a call to arms in order to fight social injustice, but there is increasing concern amongst the technical community that AI needs to be regulated in some fashion in order to prevent the proliferation of false or misleading data, and that it remains limited in terms of its control over systems that could potentially do harm.
Steps are being taken globally to discuss and deliver legislative frameworks on how to govern not only the output and reach of AI applications, but to regulate and approve the datasets that AI algorithms require to work.
Just recently a class-action lawsuit (June 2023) was filed against a well-known software company who it is alleged have been scraping personal data from the internet without permission in order to populate its massive databases. One wonders if an AI application manipulating this data that is subsequently used for a third party’s marketing purposes may become liable under this or a separate class action.
There is also the tangible concern that the very algorithms used in large language models (of the type used by generative applications such as ChatGPT) start with political or social / societal biases placed there either wittingly or unwittingly by the baseline programmers. In fact, Chat GPT suffered greatly in this regard when first launched, and still does to a degree.
Misuse, legality, and plagiarism
There have been recent documented cases of degree-level candidates utilising generative AI to compose their theses, submission papers, and reports. Just how this is dealt with by the governing bodies is currently open to question as it slips deftly through the cracks that exist between the tectonic plates of textual plagiarism, accuracy, and originality of thought.
Familiar with the “I am not a robot” question on website forms? These usually take the form of recognising an object repeated in a 12-photo matrix. An AI application recently tried to circumvent this by hiring a human on a freelancing web resource to answer the question. Further, an application that can crack software licenses is freely available to those interested in such activities. Interesting times.
There is a bright side
We can conceive of numerous practical applications of AI algorithms in manufacturing that could yield a positive and almost immediate benefit. An example may be a closed-loop CNC that adapts to SPC data measured either on or off-machine, that reads environmental parameters, and adjusts feeds & speeds, cut depths, potentially even sequencing of operations. Closed-loop feedback systems aren’t uncommon; however, they are rarely utilized to their full capability and generally won’t learn from a broader set of inputs and machine variables.
AGI has entered the “chat”
AGI, or Artificial General Intelligence is – at least currently – a hypothesis in AI’s seemingly exponential evolution. The definition of AGI describes “an autonomous system that emulates, or surpasses, that of a human in economically valuable tasks” and this places its capability at least the same as, or perhaps more advanced than, human intelligence.
AGI at this time is theoretical, and yet significant investments into this technology are being made by the biggest players in the AI arena into the hardware needed to provide the computational power, and the adaptive software necessary to drive the AI engines. AGI also represents a leap forward in capability which may render at least some of any regulatory framework obsolete.
Final thought
We are treading a fine line between political overreach, biased algorithms and governance, true potential for productivity enhancement, the inevitable reduction or even elimination of human interactions (jobs!), and highly useful data exchange and analysis.
What we can see is a huge potential to do good things, and we should exploit that potential with limited AI applications that drive our cars and machines, and program our websites. Generative AI is useful for non-critical applications but shouldn’t be utilised for core business decision-making, at least for now, unless the reference databases and AI algorithms are known and trusted.
I read recently that AGI applications are still some way from realisation because humans are currently too advanced in terms of real-world manipulation using sensory interpretation. Whilst this may be true now, I think that it misses the salient point: In my washing machine the algorithm works to clean effectively in the most environmentally friendly way possible. The inevitable conclusion over the multiple iterations necessary in order for it to achieve optimal efficiency will be to eliminate the reason that the clothes are becoming soiled in the first place……
Pacellico Blog
Embracing Sustainability: A Blueprint for Manufacturing Companies
Sustainability has become a critical focus for businesses worldwide, and the manufacturing sector is no exception. [...]
Navigating Uncertainty: Strategies for Building Resilience in Your Business Operations
No matter which business we are working in, uncertainty is the new normal. While lack [...]
The Synergy between Leadership and Management for Business Growth
In today's rapidly changing business landscape, organizations must be agile, innovative, and growth-oriented to survive [...]